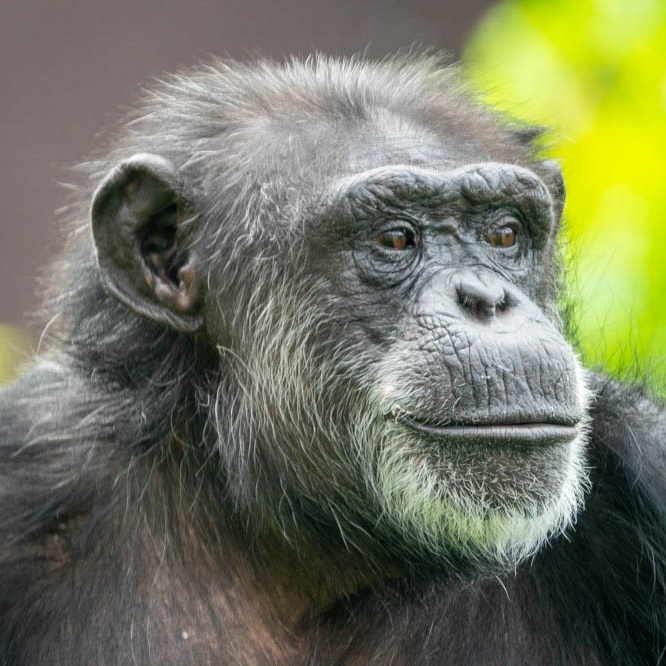
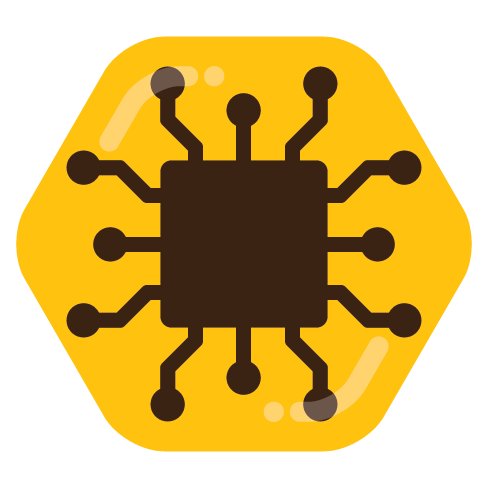
Yeah, it’s actually good. People use it even for trivial stuff nowadays; and you don’t need a pix key to send stuff, only to receive it. (And as long as your bank allows you to check the account through an actual computer, you don’t need a cell phone either.)
Perhaps the only flaw is shared with the Asian QR codes - scams are a bit of a problem, you could for example tell someone that the transaction will be a value and generate a code demanding a bigger one. But I feel like that’s less of an issue with the system and more with the customer, given that the system shows you who you’re sending money to, and how much, before confirmation.
I’m not informed on Tikkie and Klarna, besides one being Dutch and another Swedish. How do they work?
The alt text is nice, too: The weird sense of duty really good sysadmins have can border on the sociopathic, but it’s nice to know that it stands between the forces of darkness and your cat blog’s servers.